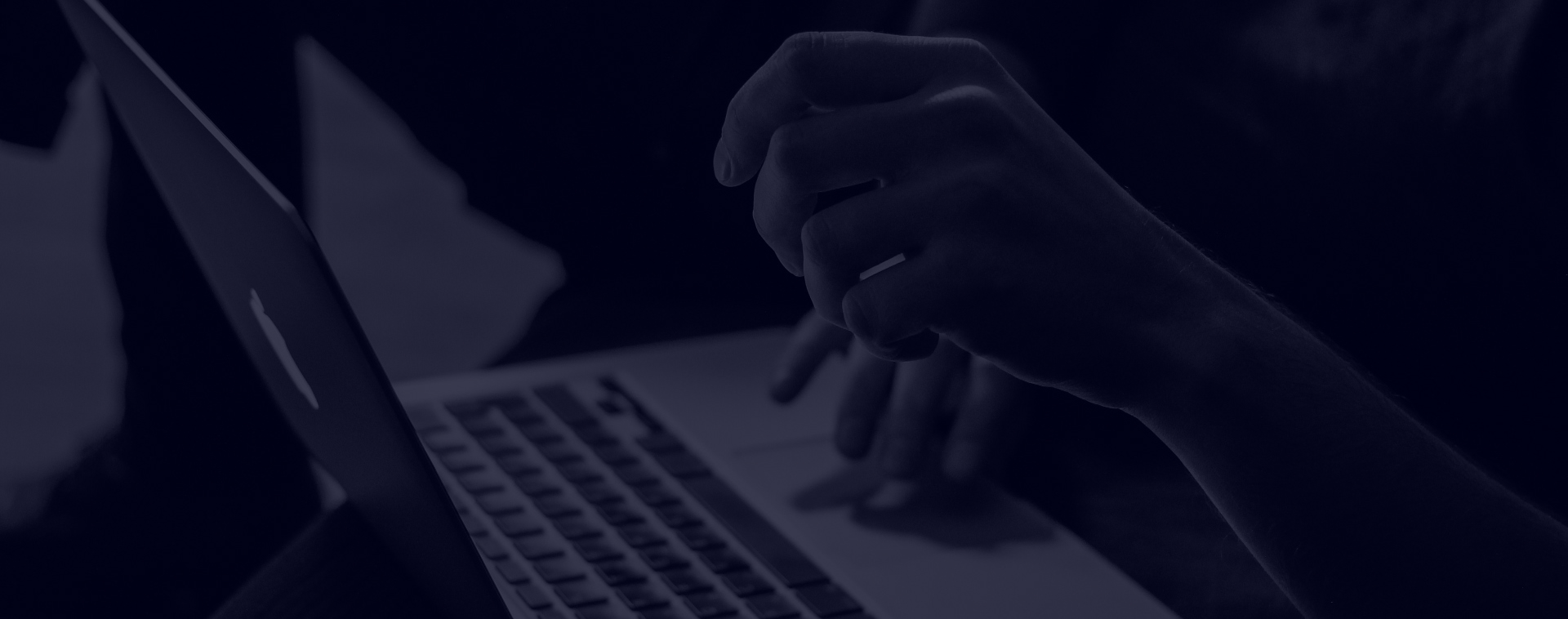
Your fully managed website,
supercharged for mobile
and search.
Blazing fast, mobile friendly web design for UK small businesses.
We handle all content updates for you, so you can focus on your business.
High performance, mobile first web design
Refresh a dated site with a design that fits your brand.
Designs are turned for mobile performance which helps your search engine ranking.
Give your website purpose
Transform your website from a simple online brochure to an engine that converts more visitors to customers.
We work with you to understand your ideal customer and re-design your site with a clear purpose or “call to action” that engages them to connect with you.
That could be anything from capturing some key information via email, setting up a discovery call or your customers picking up the phone.
How does it work?
Web design, content, hosting and cyber security. You pay nothing down and single, fixed monthly fee.
Unlimited content updates
We manage all content changes and updates for you. You no longer have to deal with frustrating or clunky content management systems.
Simply call or email, and we’ll make it happen within 24 hours. You’ll be able to preview and approve changes before they go live.
Google business listing
Help new customers find your business on Google search and Google maps.
We can review your listing and help improve your profile, advising changes to content and reviews.
Modern design with up to 10 pages.
Help new customers find your business on Google search and Google maps.
We can review your listing and help improve your profile, advising changes to content and reviews.
90%+ Google page speed score.
Last year, over 70% of UK web traffic was on mobile devices such as phones and iPads. Google is now ranking sites based on mobile site performance, but many sites are not optimised for mobile and score badly on Google’s page speed tool.
Our recent portfolio
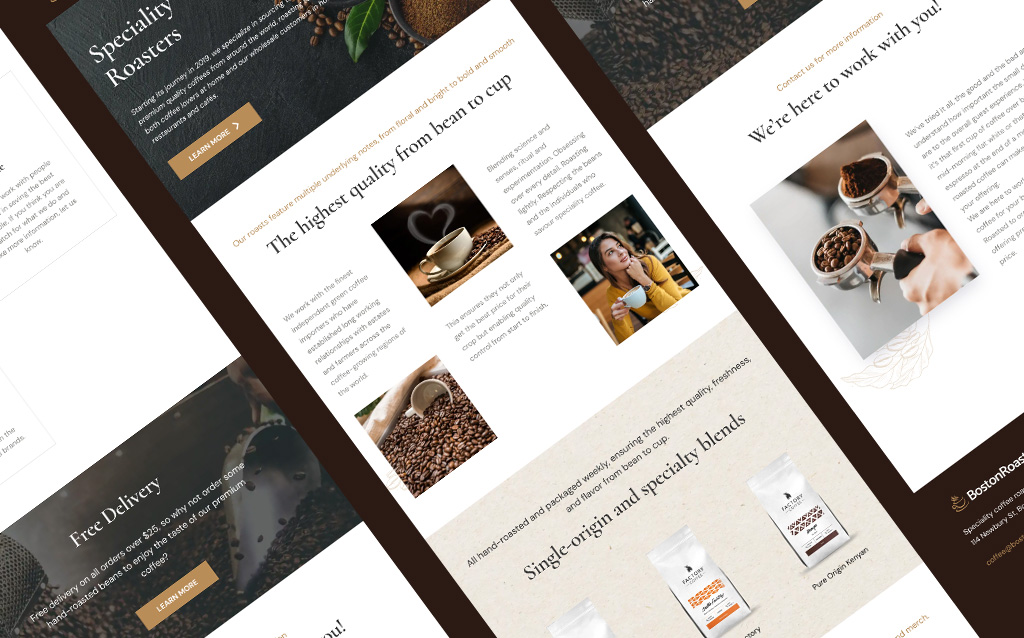
BOSTON ROASTERY
Complete redesign of the company website for their new brand. We designed the site using a rich, warm colour palette that matched their brand and reflected their premium product.
They got our full website management service including unlimited content updates and new blog pages.
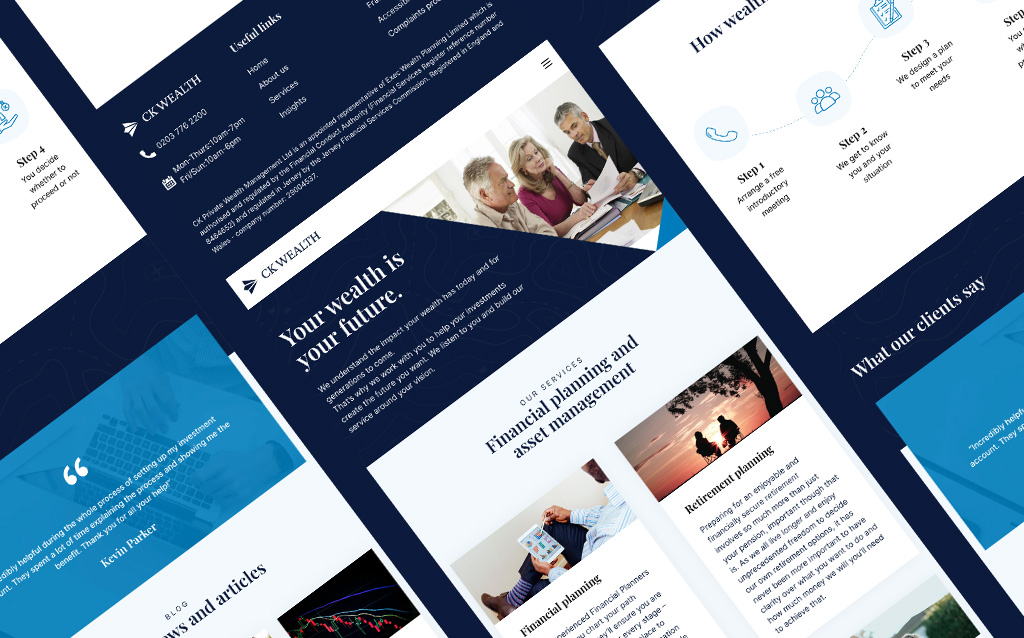
CK Weath Partners
The client was unhappy with their slow and insecure Wordpress site that was costing them a lot in ad-hoc updates and content management.
They did like their existing design, so we transferred the existing site from Wordpress to our fully managed platform. We also refreshed the content, providing a clearer call to action and added better analytics.
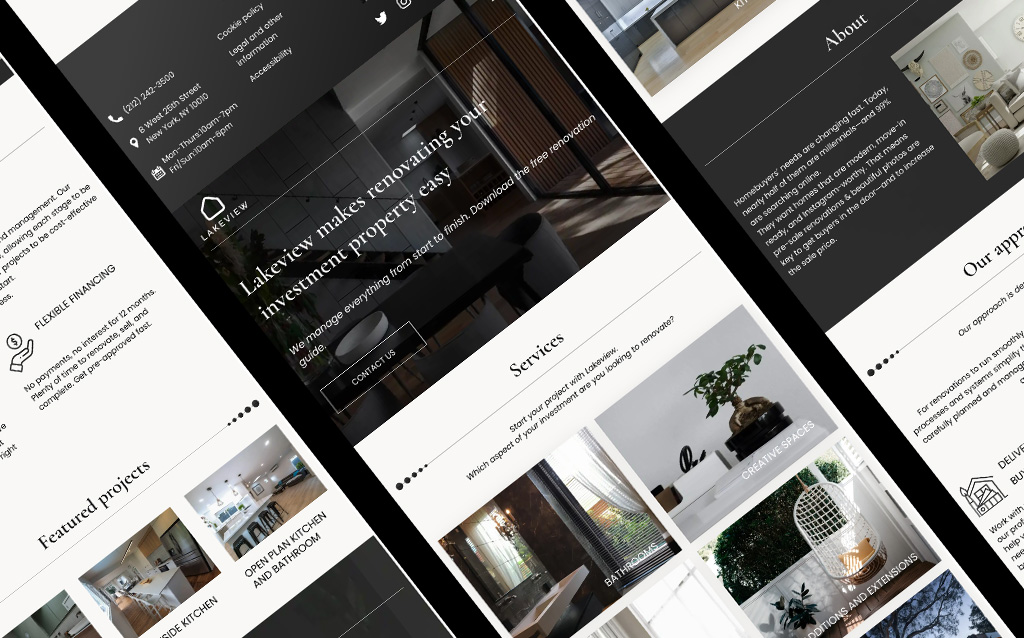
Lakeview homes
Home renovations company needed a website launched quickly. We designed a brand, designed the website, wrote the content and launched within 10 days of initial conversation.
With all the other time pressures of starting a new business, the client appreciated our fully managed content service. They don't have to worry about their website at all and trust that we "just handle it".
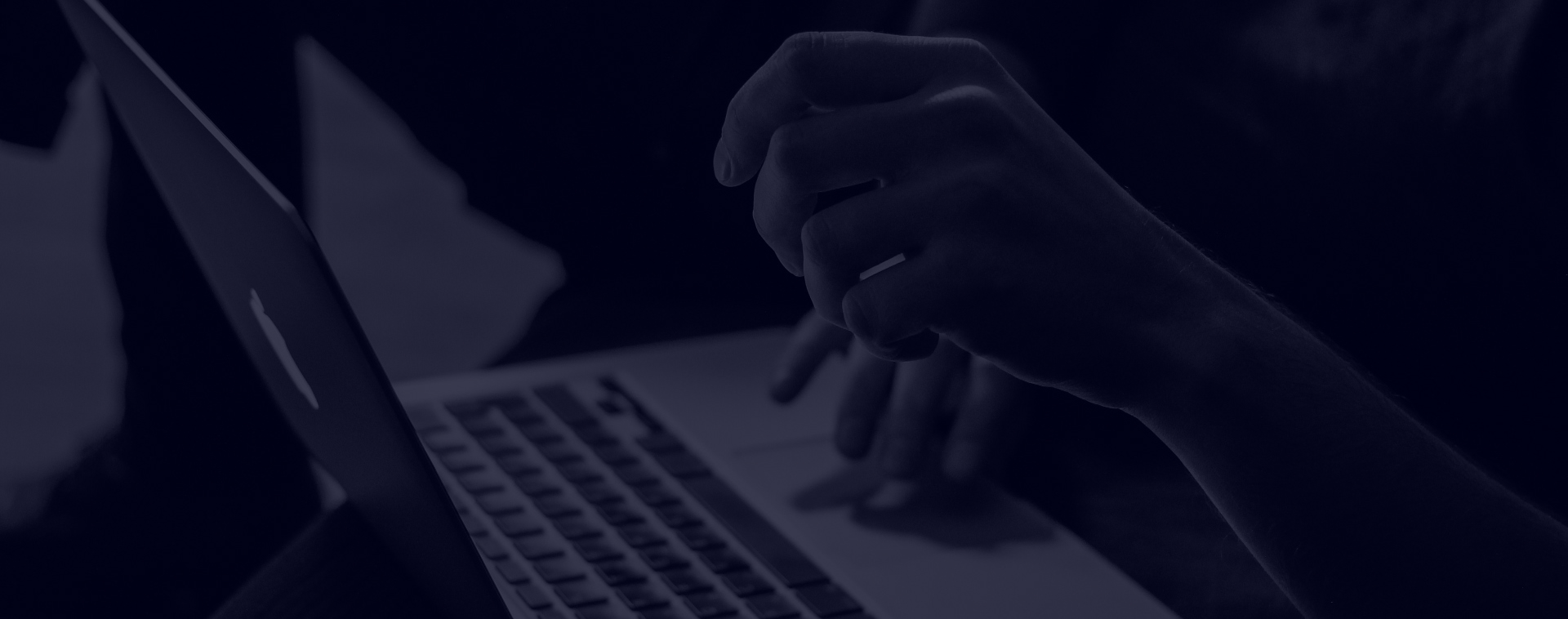
Get started
Get started for nothing down and a single, fixed monthly cost that includes 24/7 service and unlimited content changes.
[email protected]